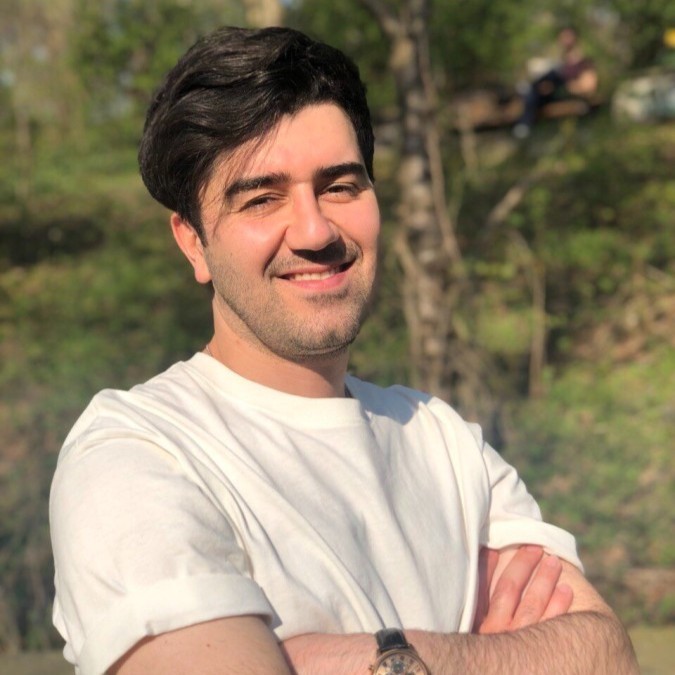
Saeed Ghoorchian
About
My research focuses mainly on building machine learning methods to solve online decision-making problems under uncertainty. To achieve this, I have worked on developing decision-making algorithms capable of learning the optimal action and feature observations in non-stationary environments with causal dependencies. Most existing machine learning methods, particularly in the era of big data, postulate the possibility of information acquisition with no limit and for free. This leads to deploying models that require observing all features' states before predicting a class label. In real-world problems, however, collecting beneficial information is often costly. For example, in online advertising problems, the advertiser can purchase information about target users to display personalized ads. As another example, in medical contexts, obtaining data for treatment recommendations mainly requires additional tests that are time- and money-consuming. How to maximize the model performance while keeping the cost of feature observations as low as possible? What if the reward distribution and features' cost distribution vary over time? Such cases frequently appear in real-world problems; for example, in personalized news recommendations, user preferences over news can change over time and exhibit various seasonality patterns. Hence, we need novel learning methods that, besides individual actions' rewards, learn the observations of the features' states and are robust to distribution shifts in the deployed environment.
Now, what if there are causal dependencies among actions' outcomes? A real-world example of this situation is the Covid-19 development within a country. During the Covid-19 pandemic, containing the virus outbreak has been one of the major concerns of governments. To this end, health authorities attempt to monitor the outbreak and detect the regions likely to become coronavirus hotspots. It is only natural that health authorities seek to find the regions that contribute the most to the total number of daily new cases in the country. Due to mobility between geographical areas, causal relations exist amongst total daily new cases (rewards) of regions (actions). How can the optimal candidate regions be detected for political interventions while dealing with statistical dependencies?
My research during my PhD addresses the questions mentioned above and the like. During my postdoc, I was doing research at the intersection of imitation learning, active learning, and inverse reinforcement learning, to design machine learning methods for explaining sequential decision-making based on demonstrated behavior by a sub-optimal learner.
Prior to my PhD, I completed my master studies in Mathematical Modelling in engineering within an Erasmus Mundus international joint master program at University of Hamburg and obtained a bachelor’s degree in Mathematics from Iran University of Science and Technology.
Research Interests
- Reinforcement Learning
- Imitation Learning
- Causality in Large Language Models
- Generative AI
- (Online) Recommender Systems
News
- June 1, 2024 I’m starting a new position as Machine Learning Scientist at SAP.
- April 2, 2024 Our paper 'Contextual Multi-Armed Bandit with Costly Feature Observation in Non-stationary Environments', has been accepted for publication at the IEEE Open Journal of Signal Processing.
- March 19, 2024 Our paper 'Non-stationary Linear Bandits with Dimensionality Reduction for Large-Scale Recommender Systems', has been accepted for publication at the IEEE Open Journal of Signal Processing.
- August 15, 2023 I joined SAP as a visiting researcher.
- April 20, 2023 I successfully defended my PhD thesis titled "Online Learning under Partial Feedback" with magna cum laude. 🎉
- September 27, 2022 I will be Teaching Assistant for the course Introduction to Game Theory with Application in Multi-Agent Systems during the winter semester at the University of Tübingen.
- July 28, 2022 Presented our accepted paper at IJCAI 2022 in Vienna.
- June 1, 2022 Joined the Decision Making group at the University of Tübingen as a research assistant.
- May 1, 2022 Starting a freelance consultant position on algorithm development at Datalyze Solutions GmbH, Germany.
- April 21, 2022 Our paper "Linear Combinatorial Semi-Bandit with Causally Related Rewards" has been accepted for publication at the 31st International Joint Conference on Artificial Intelligence (IJCAI).
- November 2, 2021 Excited to serve students as Teaching Assistant at the University of Tübingen.
- September 3, 2021 Our paper "Data-Driven Online Recommender Systems with Costly Information Acquisition" has been accepted for publication at the IEEE Transactions on Services Computing (TSC).
Publications
Saeed Ghoorchian and Setareh Maghsudi
IEEE Transactions on Cognitive Communications and Networking (TCCN), 2020
Data-Driven Online Recommender Systems with Costly Information Acquisition
Onur Atan, Saeed Ghoorchian, Setareh Maghsudi, and Mihaela van der Schaar
IEEE Transactions on Services Computing (TSC), 2021
Linear Combinatorial Semi-Bandit with Causally Related Rewards
Behzad Nourani-Koliji*, Saeed Ghoorchian*, and Setareh Maghsudi.
International Joint Conference on Artificial Intelligence (IJCAI), 2022
Bayesian Non-stationary Linear Bandits for Large-Scale Recommender Systems
Saeed Ghoorchian, Evgenii Kortukov, and Setareh Maghsudi
IEEE Open Journal of Signal Processing (OJSP), 2024
Online Learning with Costly Features in Non-stationary Environments
Saeed Ghoorchian, Evgenii Kortukov, and Setareh Maghsudi
IEEE Open Journal of Signal Processing (OJSP), 2024
Non-stationary Delayed Combinatorial Semi-Bandit with Causally Related Rewards
Saeed Ghoorchian and Setareh Maghsudi
Under review, 2023